By Ioannis Paschalidis
New Book on Distributionally Robust Learning
A central problem in machine learning is to learn from data (“big” or “small”) how to predict outcomes of interest.This learning process is vulnerable to data that may have been contaminated with outliers or are too few to accurately represent the entirety of the actual data. A new monograph develops a comprehensive statistical learning framework that is robust to (distributional) perturbations in the data using Distributionally Robust Optimization (DRO) under the Wasserstein metric. Beginning with fundamental properties of the Wasserstein metric and the DRO formulation, the book explores duality to arrive at tractable formulations and develop finite-sample, as well as asymptotic, performance guarantees. It covers a series of learning problems, including (i) distributionally robust linear regression; (ii) distributionally robust regression with group structure in the predictors; (iii) distributionally robust multi-output regression and multiclass classification, (iv) optimal decision making that combines distributionally robust regression with nearest-neighbor estimation; (v) distributionally robust semi-supervised learning, and (vi) distributionally robust reinforcement learning. A tractable DRO relaxation for each problem is being derived, establishing a connection between robustness and regularization, and obtaining bounds on the prediction and estimation errors of the solution. Beyond theory, the book includes numerical experiments and case studies using synthetic and real data. The real data experiments are all associated with various health informatics problems.
Distributionally Robust Learning provides a detailed insight into a technique that has gained a lot of recent interest in developing robust predictive and prescriptive models from data. It will be enlightening for researchers, practitioners and students working on optimization and machine learning.
The book is available from Now Publishers and Amazon.
Five Papers Detail New Computational Models to Predict Severe Illness and Mortality from COVID-19
Data Science and AI Methods Offer Predictive Systems to Aid Healthcare Policy Management and Level-of-Care Requirements
Data science and AI methods have evolved to become a powerful tool in developing strong predictive models to help improve our understanding and treatment of COVID-19. “Given ample data, and assuming that the future is not completely random (like flipping a coin), we can learn what are the specific factors influencing future outcomes and predict them with high confidence,” says CISE Director, Yannis Paschalidis, (ECE, SE, BME, CDS) .
Prof. Paschalidis brings his expertise in data science to develop models with the ability to predict the course of disease for patients who have contracted COVID-19. His work with collaborators at Boston University, Massachusetts General Hospital, Harvard Medical School, Pontifícia Universidade Católica do Rio de Janeiro, and Huazhong University of Science and Technology has resulted in five distinct papers published during 2020 in eLife, Engineering, PLOS One, JMIR Med. Informatics, and Int. J. Med. Informatics, and discoveries in the physiological and socioeconomic divide of COVID (specifically in Latin America), as well as more specific ways to predict level-of-care requirements for hospitalized COVID-19 patients in Massachusetts.
“What we found is that the course of the disease for COVID-19 patients is highly predictable,” says Paschalidis. “We now know who is more likely to be hospitalized based on age, pre-existing conditions, and basic vitals. We also know who is more likely to develop severe disease and require ICU-level care and support of their breathing through mechanical ventilation, based on their initial clinical profile upon hospital admission. COVID-19 is absolutely not your typical pneumonia or flu. It has a very distinct clinical profile.”
The team has released easy to use calculators for calculating the risk for severe COVID-19 illness for patients who have tested positive, based on demographics, pre-existing conditions, and laboratory findings.
The research also revealed that in developing countries, such as Mexico and Brazil, the socioeconomic status of the patient is an important predictor of COVID-19 outcomes, revealing existing disparities. “This is consistent with a picture that is emerging in the US as well,” says Prof. Paschalidis.
Another aspect of the research was the ability to use Artificial Intelligence to facilitate the process of processing the data to extract what is needed in training these predictive models. “In our work with data from five Massachusetts-based hospitals, we went from doctor reports all the way to predictive models using Natural Language Processing and machine learning,” adds Prof. Paschalidis. “Painstaking manual efforts to carefully compile data sets are becoming a thing of the past, drastically speeding up the process of developing models from raw data.”
Learn more about the papers here:
Boran Hao, Shahabeddin Sotudian, Taiyao Wang, Tingting Xu, Yang Hu, Apostolos Gaitanidis, Kerry Breen, George C Velmahos, Ioannis Ch Paschalidis (Boston University, Massachusetts General Hospital, Harvard Medical School), “Early prediction of level-of-care requirements in patients with COVID-19,” eLife, Oct. 12, 2020, doi: 10.7554/eLife.60519.
Overview: This study examined records of 2,566 consecutive COVID-19 patients at five Massachusetts hospitals and sought to predict level-of-care requirements based on clinical and laboratory data. Several classification methods were applied and compared against standard pneumonia severity scores. The need for hospitalization, ICU care, and mechanical ventilation were predicted with a validation accuracy of 88%, 87%, and 86%, respectively. ICU care and ventilation. When predictions are limited to patients with more complex disease, the accuracy of the ICU and ventilation prediction models achieved accuracy of 83% and 82%, respectively. Vital signs, age, BMI, dyspnea, and comorbidities were the most important predictors of hospitalization. Opacities on chest imaging, age, admission vital signs and symptoms, male gender, admission laboratory results, and diabetes were the most important risk factors for ICU admission and mechanical ventilation. The factors identified collectively form a signature of the novel COVID-19 disease.
Salomón Wollenstein-Betech, Amanda A. B. Silva, Julia L. Fleck, Christos G. Cassandras, Ioannis Ch. Paschalidis (Boston University, Pontifícia Universidade Católica do Rio de Janeiro), “Physiological and socioeconomic characteristics predict COVID-19 mortality and resource utilization in Brazil,” PLOS ONE, Oct. 14, 2020, https://doi.org/10.1371/journal.pone.0240346.
Overview: This study used a nation-wide multicenter data of COVID-19 patients in Brazil to predict mortality and ventilator usage. A total of 113,214 patients with 50,387 deceased, were included. Death from COVID-19 was strongly associated with demographics, socioeconomic factors, and comorbidities. Classifying the medical risk of COVID-19 patients was shown to be relevant for low- and medium- income countries in order to assign limited medical resources more effectively, as well as to help design targeted physical-distancing and work accommodation policies that will assist in reducing economic loss during the current pandemic. The results highlight the predictive power of socioeconomic information in assessing COVID-19 mortality and medical resource allocation, and shed light on existing disparities in the Brazilian health care system during the COVID-19 pandemic. In the future, this model could help prioritize vaccine distribution to the more risk-vulnerable and to those who need to interact with them.
Salomón Wollenstein-Betech, Christos G. Cassandras, Ioannis Ch. Paschalidis (Boston University), “Personalized Predictive Models for Symptomatic COVID-19 Patients Using Basic Preconditions: Hospitalizations, Mortality, and the Need for an ICU or Ventilator,” International Journal of Medical Informatics, Volume 142, October 2020, 104258, https://doi.org/10.1016/j.ijmedinf.2020.104258.
Overview: This study developed personalized models that predict an individual’s likelihood of hospitalization, mortality, need for ICU, and need for ventilation with a sample of 91,000 COVID-19 cases from records made public by the Mexican government. After analyzing the information, the researchers built models that consider pre-existing conditions and how the disease could progress. They discovered that key factors affecting the need for hospitalization and ICU included age, pregnancy, diabetes, gender, chronic renal insufficiency, and immunosuppression.
Taiyao Wang, Aris Paschalidis, Quanying Liu, Yingxia Liu, Ye Yuan, and Ioannis Ch. Paschalidis (Boston University, Brown University, University of Science and Technology, Shenzen, Huazhong University of Science and Technology, Wuhan), “Predictive Models of Mortality for Hospitalized COVID-19 Patients: Retrospective Cohort Study,” JMIR Medical Informatics, Vol. 8, No. 10, e21788, 2020, https://doi.org/10.2196/21788.
Overview: This study developed accurate models to predict the mortality of hospitalized patients with COVID-19 using basic demographics and easily obtainable laboratory data, analyzing data for 375 hospitalized patients with COVID-19 in Wuhan, China. Two types of models were developed: the first type used laboratory findings from the entire length of the patient’s hospital stay, and the second type used laboratory findings that were obtained no later than 12 hours after admission. The models were further validated on a multicenter external cohort of 542 patients. The models achieved high accuracies and could predict outcomes more than one week in advance; suggesting that they can be highly useful for resource allocation in hospitals.
YeYuan, Chuan Sun, Xiuchuan Tang, Cheng Cheng, Laurent Mombaerts, Maolin Wang, Tao Hue, Chenyu Sun, Yuqi Guo, Xiuting Li, Hui Xu, Tongxin Ren, Yang Xiao, Yaru Xiao, Hongling Zhu, Honghan Wu, Kezhi Li, Chuming Chen, Yingxia Liu, Zhichao Liang, Zhiguo Cao, Hai-Tao Zhang, Ioannis Ch. Paschalidis, Quanying Liu, Jorge Goncalves, Qiang Zhong, and Li Yan (Huazhong University of Science and Technology, Wuhan, University of Luxembourg, University of Cambridge, AMITA Health Saint Joseph Hospital Chicago, University College London, Southern University of Science and Technology, Shenzhen, Boston University), “Development and Validation of a Prognostic Risk Score System for COVID-19 Inpatients: A Multi-Center Retrospective Study in China”, Engineering, 2020, https://doi.org/10.1016/j.eng.2020.10.013.
Overview: This study developed a risk score using clinical data from 1479 inpatients admitted to Tongji Hospital, Wuhan, China (development cohort) and externally validated with data from two other centers: 141 inpatients from Jinyintan Hospital, Wuhan, China (validation cohort 1) and 432 inpatients from The Third People’s Hospital of Shenzhen, Shenzhen, China (validation cohort 2). The risk score is based on three biomarkers that are readily available in routine blood samples and can easily be translated into a probability of death. The risk score can predict the mortality of individual patients more than 12 days in advance with more than 90% accuracy across all cohorts.
New COVID-19 research focuses on Latin America
Informing Policy, Resource Allocation and Workplace Adjustment Policies
COVID-19 has taken the world by storm, placing significant pressures on healthcare systems. Particularly in countries with limited testing resources and capacity-constrained health care systems, it is essential to determine who is at most risk for developing COVID-19. Knowing who may or may not need medical attention, and what type of medical attention enables planning and resource allocation at the local, state, and nationwide level.
To address this need, researchers from Boston University College of Engineering and the Center for Information and Systems Engineering (CISE) conducted a study to predict COVID-19 outcomes in Mexico using Machine Learning methods. Study results were published today in the International Journal of Medical Informatics in a paper titled “Personalized Predictive Models for Symptomatic COVID-19 Patients Using Basic Preconditions: Hospitalizations, Mortality, and the Need for an ICU or Ventilator.”
Developing Risk Assessment Models for Patient Outcomes
Yannis Paschalidis, CISE Director and Professor (ECE, SE, BME, CDS), Christos Cassandras, Head of the Division of Systems Engineering, and Professor (ECE, SE), and Salomón Wollenstein-Betech, Ph.D. candidate (SE), developed personalized models that predict an individual’s likelihood of hospitalization, mortality, need for ICU, and need for ventilation with a sample of 91,000 COVID-19 cases from records made public by the Mexican government. After analyzing the information, the researchers built models that consider pre-existing conditions and how the disease could progress. They discovered that key factors affecting the need for hospitalization and ICU included age, pregnancy, diabetes, gender, chronic renal insufficiency, and immunosuppression.
“The virus appears to be basically the same around the world; still societies get impacted in very different ways, not only because of local and national policies but also because of different demographics and prevalence of underlying diseases,” says Paschalidis. “This work enabled us to focus on Mexico, for which not much work had been done, and develop custom models that take into account local characteristics.”
The idea [for the paper] was not to build an accurate clinical decision support tool; rather the objective was to do risk assessment to inform policy and resource allocation,” explains Wollenstein-Betech. “In particular, in countries like Mexico or other low-medium income countries, COVID has been very taxing on the health care system. Our risk assessment allows individuals to assess their own risk without going to a hospital and getting tested… so the government can assign resources to treat people who need it the most.”
The BU team used a larger data set than previous predictive models, therefore reducing bias and increasing accuracy. Said models determined by Paschalidis, Cassandras, and Wollenstein-Betech achieved a 72% accuracy for predicting hospitalization, 79% for mortality, 89% for ICU admission, and 90% for use of a ventilator.
“This is a first among a series of our work on COVID-19, utilizing data and employing data science methods to predict who is at risk for more severe versions of the disease,” says Paschalidis.
Cassandras adds that “Models of this type are extremely useful for resource allocation and staffing at hospitals, but also to inform workplace adjustment policies and prioritize vaccination when a vaccine becomes available.”
With further research, the models could spearhead new developments in policies and guidelines to better protect vulnerable populations. Read the paper here.
By Eliza Shaw, CISE Staff
AI in Medicine Advances with BU-MIT Research Team
Researchers from Boston University and the Massachusetts Institute of Technology have pioneered an AI method that learns from existing data how to make specific recommendations (“prescriptions”) to optimize a certain outcome. Their research findings have been published in this month in PLOS ONE.
The paper titled “Prescriptive Analytics for Reducing 30-day Hospital Readmissions after General Surgery” proposes an innovative solution for reducing re-hospitalization rates. Combining predictive and prescriptive statistical modeling, researchers Ioannis (Yannis) Paschalidis and Taiyao Wang of Boston University, and Dimitris Bertsimas and Michael Lingzhi Li of MIT, identify the factors behind re-hospitalization rates and suggest an effective treatment: targeted preoperative blood transfusions.
“The vast majority of AI in medicine develops models that learn how to predict some outcome,” says Dr. Paschalidis, Professor of the College of Engineering (ECE, BME, SE, CDS) and Director of the Center for Information & Systems Engineering at BU. “What is novel in this work is that we train a model that offers personalized recommendations/prescriptions aimed at improving patient outcomes. The approach has broad applicability and the potential to improve medical decision making.”
The method results from thorough research and application. Using data from more than 700,000 surgeries from the American College of Surgeons National Surgical Quality Improvement Program (NSQIP), the researchers analyzed how pre-operative factors (such as underlying medical conditions) and post-operative factors (including complications from surgery) affect current re-hospitalization rates.
By incorporating both pre-operative and post-operative factors in their prescriptive models, the researchers discovered pre-operative hematocrit levels significantly affected re-hospitalization rates. From there, they created a prescriptive model that suggested targeted pre-operative transfusions to increase hematocrit. As a result, the blood transfusions reduced the likelihood of a re-hospitalization.
As it stands, the U.S. spends over $3 trillion annually on healthcare, with a large portion resulting from re-hospitalization rates. However, with the presented research, that number could fall. The researchers estimate a 12% decrease in 30-day rehospitalization rates if one were to follow the model, potentially saving the U.S. over $20 million on an annual basis.
“The use of AI in medicine is likely to increase, helping to improve patient outcomes and reduce costs,” adds Dr. Paschalidis. “This paper is an example of how AI could be used to affect modifiable factors that improve outcomes through personalized medicine.”
To learn more about the research and methods of this publication, read the full paper here.
PLOS ONE is the world’s first multidisciplinary Open Access journal. The journal’s publication criteria are based on high ethical standards and the rigor of the methodology and conclusions reported.
By Maria Yaitanes, CISE Staff
Is Artificial Intelligence A Blessing Or A Curse For The Environment?
“In the past few years, as AI usage has grown, its energy consumption and carbon emissions have become an environmental concern,” writes Ben Dickson, a software engineer, tech blogger, in PC Magazine.
Yannis Paschalidis, Director of Boston University’s Center for Information and Systems Engineering, is featured in the story, discussing ways to create energy-efficient AI hardware.
Prof. Paschalidis proposes a better integration of data centers and energy grids, which he describes as “demand-response” models. ‘The idea is to coordinate with the grid to reduce or increase consumption on-demand, depending on electricity supply and demand. This helps utilities better manage the grid and integrate more renewables into the production mix… The smart integration of power grids and AI data centers, Paschalidis says, will help manage the intermittency of renewable energy sources while also reducing the need to have too much stand-by capacity in dormant electricity plants.”
Read the full PC Magazine story here.
Changing the Ways of Selfish Driving
Salomón Wollenstein-Betech (PhD candidate, SE) develops dynamic, data-driven tools to estimate and optimize congested roadways
Boston has the worst traffic in the nation, according to a new study released this week. As city traffic thickens, the quintessential need for a resolution to this problem arises.
Boston University PhD candidate (Systems Engineering) Salomón Wollenstein-Betech has created a visual interactive data-driven platform that tracks annual traffic conditions in the Eastern Massachusetts (EMA) area. Titled Congestion Maps, the tool proves to be a well-needed analysis to inform urban planners, policy makers, researchers, and the general public the conditions of a transportation network over time. This knowledge will help to focus on where to optimize the transportation network by identifying bottlenecks and the most affected areas.

Originally from Mexico City, ranked the third the most traffic-congested city on the planet, Wollenstein-Betech fully appreciates the challenges associated with heavy urban traffic. “The complexity of living in one of the biggest cities in the world, such as Mexico City, makes it interesting to solve problems involved with traffic congestion, water management, and electricity,” says Wollenstein-Betech. “As city population increases, the amount of problems to tackle does as well.”
Wollenstein-Betech arrived at the BU Center for Information and Systems Engineering (CISE) in 2017 after receiving his B.S. in Industrial Engineering from the Tecnológico de Monterrey, a private research university in Mexico. Centering his research efforts alongside his advisors, Professors Christos Cassandras and Yannis Paschalidis, he is primarily interested in systems engineering at the intersection of operations research, engineering, optimization, and society. He draws inspiration on tackling complex social problems by the use of optimization and machine learning from his academic studies, his post-college professional experience, and by growing up in Mexico City, a highly dynamic metropolis.
“With the emergence of self-driving cars and smart cities, analyzing traffic data is becoming fundamental for making smarter decisions,” says Wollenstein-Betech.
As ride-sharing apps increase in popularity and autonomous vehicles are soon to be introduced, Wollenstein-Betech sees them “changing the dynamics of the streets and modifying the way humans and vehicles interact with the city resources.” He also mentions that “instead of driving selfishly, we want to route individuals jointly in order to minimize their aggregated travelling time and maximize the network efficiency.” Research shows that traffic routing apps, like Waze, Google Maps, and Apple Maps are not optimizing the overall network as they optimize each driver selfishly, and can even worsen traffic conditions. Wollenstein-Betech aims to change that.
His visualization tool is different than current mapping technologies because it estimates the traffic congestion on a road network, rather than reporting road speed as Google Maps does. He describes it as a first step to figure out the larger problem of traffic congestion and transportation.
Wollenstein-Betech uses speed data that was made available by the Central Transportation Planning Staff (CTPS) of the Boston Metropolitan Planning Organization to pinpoint specific locations where traffic condition deterioration is at its worst. This allows users to compare travel time and congestion in the EMA network in 2012 and 2015 by road segment and zip code. The data gives researchers, like Wollenstein-Betech, an opportunity to inform policy makers to consider specific strategies when targeting more congested areas in the network.
“There’s a lot that could be done with this information,” says Wollenstein-Betech. “It lends itself to new avenues relating to ride-sharing, smart parking, congestion tolling, and lane reversal.” Wollenstein-Betech is excited to see where this research will take him next.
In his free time, Wollenstein-Betech avoids traffic by spending time outside and away from the busy roads. He enjoys outdoor adventures and loves the four seasons of New England. “Winter is too long though in Boston,” he says with a laugh. “But it’s interesting to live in such a dynamic place in terms of weather.”
By Allie Antonevich, CISE Staff
Yannis Paschalidis Named the 2020 Charles DeLisi Distinguished Lecturer
Professor Yannis Paschalidis is the 2020 recipient of the Charles DeLisi Award and Distinguished Lecture. This award celebrates high-impact research in engineering. This showcasing event allows all members of the Boston University community to meet a distinguished scholar selected from the College of Engineering faculty discussing a topic of recognized excellence.
Professor Paschalidis will present on “Data Science and Optimization Adventures in Computational Biology and Medicine.” In this lecture, he will present several seemingly disparate areas of his work in computational biology and medicine connected through the use of data science and optimization methods. Topics range from the molecular to the whole organism/disease level and models progress from predictive to prescriptive. View the abstract. The date and time of the presentation has been posted from April 27th, 4PM, to a date and time to be announced later.
Yannis Paschalidis is a Professor and Data Science Fellow in Electrical and Computer Engineering, Systems Engineering, Biomedical Engineering, and Computing & Data Sciences at Boston University. He is the Director of the Center for Information and Systems Engineering (CISE), a university-wide interdisciplinary research center at Boston University administered by the College of Engineering.
Professor Paschalidis has distinguished himself as a researcher, scholar, and educator whose work spans the fields of systems and control, optimization, networks, operations research, computational biology and medical informatics. Dr. Paschalidis’ research has impacted a breadth of applications, including predictive health analytics, protein docking, autonomous robots, sustainable energy, and smart cities, among others.
At BU since 1996, Dr. Paschalidis has authored more than 200 refereed publications with his students and collaborators from diverse disciplines. He has been a PI or Co-PI on numerous interdisciplinary grants totaling more than $43 million and has advised 24 Ph.D. theses.
Prof. Paschalidis’ work has been recognized with a CAREER award from the National Science Foundation, the second prize in the 1997 George E. Nicholson paper competition by INFORMS, the best student paper award at the 9th Intl. Symposium of Modeling and Optimization in Mobile, Ad Hoc, and Wireless Networks (WiOpt 2011) won by one of his Ph.D. students for a joint paper, an IBM/IEEE Smarter Planet Challenge Award, and a finalist best paper award at the IEEE International Conference on Robotics and Automation (ICRA). His work on protein docking (with his collaborators) has been recognized for best performance in modeling selected protein-protein complexes against 64 other predictor groups (2009 Protein Interaction Evaluation Meeting). His recent work on health informatics won an IEEE Computer Society Crowd Sourcing Prize and a best paper award by the International Medical Informatics Associations (IMIA). He was an invited participant at the Frontiers of Engineering Symposium organized by the National Academy of Engineering, and at the 2014 National Academies Keck Futures Initiative (NAFKI) Conference. Prof. Paschalidis is a Fellow of the IEEE and the founding Editor-in-Chief of the IEEE Transactions on Control of Network Systems.
Paschalidis received a Diploma (1991) from the National Technical University of Athens, Greece, and an M.S. (1993) and a Ph.D. (1996) from the Massachusetts Institute of Technology (MIT), all in Electrical Engineering and Computer Science.
By Maria Yaitanes, CISE Staff
Moths Teach Drones to Fly
Research is first to apply animal data to autonomous vehicle navigation
By Liz Sheeley
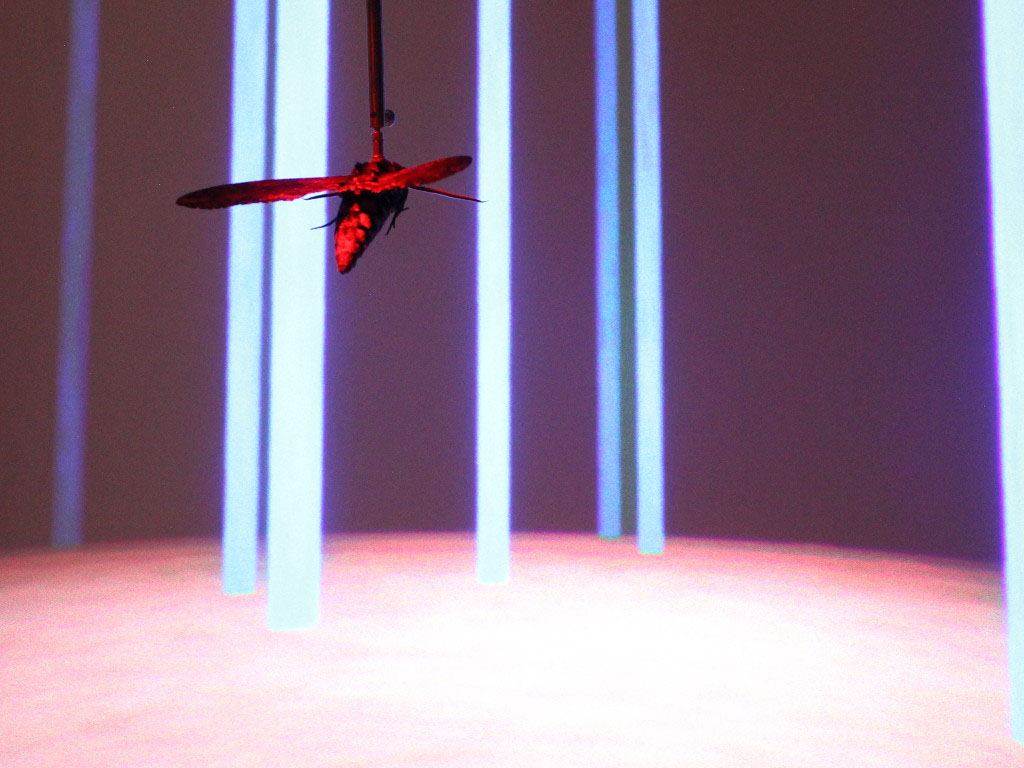
When an autonomous drone is deployed for a mission, it flies on a specific, programmed route. But if there are any surprises along the way, the drone has a difficult time adapting to a change because it hasn’t been programmed on how to do that.
Now, researchers have been able to develop a new paradigm that would allow a drone to fly from point A to point B without a planned route, with the help of moths. Professor Ioannis Paschalidis (ECE, SE, BME), his team and collaborators at the University of Washington have been able to extract information about how a particular species of moth travels through a forest and then use that data to create a new control policy for drones. Their work has been published in PLoS Computational Biology and it is the first published work that uses data from an animal to improve autonomous drone navigation.
They first did this by having the researchers at the University of Washington collect data from how the moths would fly around a virtual forest, taking measurements about things like their flight trajectories, force and speed along the way. Those data were then used to extrapolate what kind of navigation policy the moths used.
“We discovered that the moths relied heavily on what is known as optical flow,” says Paschalidis. “That is a pattern of the motion of the various objects in the environment that is caused by your own motion relative to these objects, in this case the moth’s own motion.”
That perceived motion then allows the moth to react to objects in the appropriate way, as objects that are moving fast are close, and those that are further are moving slowly. This is similar to how humans react while driving; if a car suddenly appears extremely fast in front of your car, you’ll brake, but if a car is far away and speeding around, then you don’t have to react quite as quickly.
Understanding how the moths fly helped the researchers develop a drone policy that was then tested on virtual drones as they flew through a virtual forest. To see how robust this policy was, the researchers had the drones fly through multiple types of environments—from a complex, heavily wooded forest to a sparse one.
Paschalidis says the most surprising element of this study is that the policy helped the drone navigate through all of the different environments.
Typically, drone control policies are optimized for a specific mission, which renders them fragile to unexpected events. This research is getting one step closer to an adaptive, self-aware autonomous vehicle policy so it can fly in a dynamic, complex and unknown environment.
The work is part of a $7.5 million multidisciplinary university research initiative (MURI) grant awarded by the Department of Defense for developing neuro-inspired autonomous robots for land, sea and air.
Paschalidis says that collaborators from other universities will help gather data from other animals and insects such as mice and ants to develop more robust autonomous vehicle navigation policies.
Originally published on BU College of Engineering News on February 3, 2020.
PLOS Computational Biology: Learning from Animals: How to Navigate Complex Terrains
PLOS Computational Biology issued a press announcement on a paper that it published today authored by Boston University CISE Director Yannis Paschalidis (Professor ECE, SE, BME), PhD candidate Henghui Zhu (SE), former CISE post-doctoral associate Armin Ataei, former CISE visiting student scholar Hao Liu (Zhejiang University), along with University of Washington collaborators Professor Thomas Daniel (BIO, NEUROSCI) and postdoctoral researcher Yonatan Munk (BIO).

The paper, entitled Learning from Animals: How to Navigate Complex Terrains, includes findings from the study of hawkmoths’ flight data that could be applied to develop decision-making programs for autonomous vehicles.
In the study, the researchers analyzed data from hawkmoth flight trajectories in a closed-loop virtual forest and extracted and adapted the navigation control policy for autonomous vehicles. Among key findings, the study revealed that moths rely heavily on optical flow (the pattern of apparent motion of objects in a scene caused by the animal’s movement) to navigate rather than explicitly observing the location of the various obstacles in the scene. The researchers also found that the navigation strategy of moths could be adapted to design control policies guiding the movement of drones in cluttered environments.
“This work opens the door to learning from animals and humans how to perform certain tasks using autonomous robots,” says Prof. Paschalidis. “The study analysis could help synthesize novel navigation control strategies for autonomous vehicles operating in similar environments.”
Bio-inspired navigation is an area of focus for Boston University researchers as underscored by a multitude of research initiatives, including the recently won ONR MURI led by Prof. Paschalidis entitled: Neuro-Autonomy: Neuroscience-Inspired Perception, Navigation, and Spatial Awareness for Autonomous Robots.
Read the article “Learning from Animals: How to Navigate Complex Terrains” in PLOS Computational Biology 16(1): e1007452 here: doi.org/10.1371/journal.pcbi.1007452
Read related articles:
“Aerial Drones Get Schooled in Navigation“, The Brink, by Kerry Benson
“Moths Teach Drones to Fly“, BU College of Engineering News, Liz Sheeley
“Flight Data of Moths Used to Help Drones Fly Better“, Courthouse News, by Nathan Solis
Press inquires, please contact Maureen Stanton at CISE. Phone: (617) 358-5973. Email: stanton@bu.edu
BU-Harvard Team Wins $1.2M NSF Grant to Improve Women’s Reproductive Health using AI and Machine Learning

Researchers to advance distributed analytics to enhance fertility in families
A multidisciplinary team of researchers from Boston University and Harvard University is working to address women’s reproductive health challenges with the help of a $1.2M, four-year grant funded by the National Science Foundation (NSF) through its Smart and Connected Health (SCH) program. The BU-led project will leverage machine learning and artificial intelligence to develop an integrative approach to enable personalized reproductive/fertility predictions and individualized prescriptions to help address fertility problems. The researchers will also focus on improving the understanding of socioeconomic disparities in the use of infertility treatment services.
The demands of modern life, education and career choices, as well as the availability of assisted reproductive technologies, are leading many individuals and couples to delay childbearing. This has contributed to infertility and sub-fertility emerging as significant public health problems in the U.S., affecting about 15% of couples, and resulting in more than $5 billion spent annually in infertility services. Such costs are often not covered by health insurance and, consequently, generate access disparities.
“This project is an exciting illustration of the tremendous potential modern data science methods have to leverage the increasing availability of data and impact our health and wellbeing,” says Boston University College of Engineering Professor Ioannis (Yannis) Paschalidis, principal investigator (PI) of the project and director of the Center for Information and Systems Engineering. “With the emergence of personalized medicine, aided by data and algorithmic advances, we now have the ability to learn from available data and develop personalized predictions and intervention recommendations for each individual. The overall goal is to enable people to optimize health before conception, identify modifiable determinants of fertility, and reduce health risks during pregnancy and beyond.”
Innovative team of engineers and clinicians bring a synergistic range of expertise
The BU-Harvard University research team reflects the integrative nature of the project, bringing together algorithms, public health, and medical expertise to address clinical and socio-economic challenges contributing to infertility and women’s reproductive health.

The BU team of principal investigators include: Yannis Paschalidis, BU Professor of Electrical and Computer Engineering, Systems Engineering, and Biomedical Engineering, an expert in decision theory, networks, machine learning, optimization, and computational biology/medicine; Alex Olshevsky, BU Professor of Electrical and Computer Engineering and Systems Engineering, an expert in distributed optimization methods; and Lauren Wise, ScD, BU Professor of Epidemiology, BU School of Public Health, an expert in reproductive epidemiology and PI of the BU Pregnancy Study Online (PRESTO).
Harvard University is the subaward recipient on this grant, and brings the expertise of Co-PI Shruthi Mahalingaiah, Assistant Professor of Environmental Reproductive and Women’s Health. Professor Mahalingaiah is a reproductive endocrinologist, infertility physician scientist and PI of the Ovulation/Menstruation Health Study. Dr. Mahalingaiah holds an adjunct appointment at the BU School of Medicine in the Department of OB/GYN and directs the Program in Environment and Women’s Health, a multi-institutional collaborative effort which includes Boston Medical Center, BU School of Medicine, BU College of Engineering (Paschalidis), and Massachusetts General Hospital, and is housed at the Harvard T.H. Chan School of Public Health.
A shifting paradigm in health data collection
Electronic Health Record (EHR) data are distributed at many locations, hospitals, doctor offices, and other clinics. Medical devices are also becoming smaller with the ability to transmit and store information at home monitoring stations, a patient’s smartphone, and the cloud.
“While traditional learning methods require collecting all data at a central location, this is becoming increasingly harder, even undesirable for privacy reasons,” says Co-PI Alex Olshevsky. “Clearly, the more locations contain sensitive data, the greater is the likelihood of a data breach. In this context, it becomes important to develop fully distributed algorithms that can provide privacy guarantees.”
The researchers will develop an integrative approach to enable personalized reproductive/fertility predictions and prescriptions using distributed, privacy-preserving algorithms trained using multiple data sources. The algorithms will combine information from self-administered surveys, electronic health records, and personal health records to produce highly accurate personalized predictions and prescriptions or recommendations, enabling individuals and their physicians to make the most appropriate, individualized health care decisions.
“Collaboration for improving discovery and improving care for women across the lifecourse is critically important,” says Dr. Mahalingaiah. “Merged datasets including self reporting, lifestyle, and exposures, clinical-grade data, and data collected from wearable devices will provide personalized insights so that women can be empowered to understand information on the health of their bodies and make the best choices for their health and futures.”
Predictive fertility/family planning models
The research team will develop fertility/family planning models, including predicting pregnancy, the success of an IVF cycle, the presence of specific reproductive health issues affecting fertility, and making related health care recommendations. Predictive models will identify the most important factors associated with reduced fertility or Assisted Reproductive Technologies (ART) success rates, which could pinpoint specific lifestyle habits, environmental factors, and other key drivers of reduced positive outcomes that can inform health policy recommendations. A key focus will be on ovulation disorders, including Polycystic Ovary Syndrome, which are the leading cause of female infertility and are associated with an increased risk of chronic diseases, such as diabetes and cardiovascular disease.
“Machine learning has the potential to identify novel determinants of subfertility in large prospective cohort studies like PRESTO,” says Dr. Wise. “Developing tools for individuals to quantify their probability of conception based on personal inputs is paradigm-shifting. We are delighted to be partnering with Dr. Paschalidis and his colleagues on this innovative study.”
Professor Paschalidis adds, “It’s one thing to be able to predict one thing will happen, but the important question is, what do you do? How do you improve the condition of the individual? By bringing together this truly multidisciplinary collaboration of clinicians and engineers, this project brings the deep, diverse expertise and requisite resources for advancing the health and wellbeing of women.”
By Maureen Stanton, CISE