Big Data Improving Health Care
Data scientist and physician team up to reduce preventable hospitalizations
By Suzanne Jacobs, BU Research
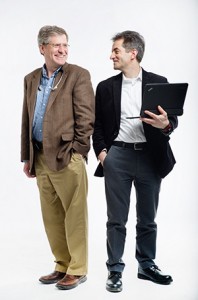
Big Data Meets Healthcare: Bill Adams, a physician and medical informatician, and Yannis Paschalidis, a data scientist and engineer, are working together to use data from electronic health records to reduce preventable hospitalizations and cut health care costs. Photo by Jackie Ricciardi
Big Data Meets Healthcare: Bill Adams, a physician and medical informatician, and Yannis Paschalidis, a data scientist and engineer, are working together to use data from electronic health records to reduce preventable hospitalizations and cut health care costs. Photo by Jackie Ricciardi
Professor Yannis Paschalidis (ECE, SE, BME), a data scientist, has built a career on making things run smoothly and efficiently—transportation systems, communication networks, supply chains, sensor networks—and now he’s taking on perhaps his most ambitious challenge yet: the US health care system.
It all started about three years ago. Paschalidis, a Distinguished Faculty Fellow at Boston University’s College of Engineering (ENG), read in a study by the US Department of Health and Human Service’s Agency for Healthcare Research and Quality (AHRQ) that in 2006, the US spent about $30.8 billion on hospitalizations that could have been prevented through better patient care, healthier patient behavior, or improved ambulatory services.
“I was reading a lot of things about the sorry state of the health care system in the US and how inefficient it is, and I thought it’s an opportunity to do something,” says Paschalidis, who also directs BU’s Center for Information & Systems Engineering. “I thought people like me that have a quantitative, more optimization-oriented background could contribute something.”
And so, having never worked in medicine before, Paschalidis teamed up with William G. Adams, a Boston Medical Center (BMC) physician and BU School of Medicine professor of pediatrics. With a team of graduate students and nearly $2 million from the National Science Foundation, the two set out to build a piece of software that could automatically flag patients at increased risk for medical emergencies by using data from their electronic health records (EHRs). They decided to start with heart diseases, which alone cost the US more than $9.5 billion in preventable hospitalizations in 2006, according to the AHRQ study.
To understand how Paschalidis works, think of how an autopilot controls an airplane. As a plane flies, autopilot software takes in data about its position and uses that data to adjust the plane’s trajectory as necessary. It’s a constant flow of data intake, analysis, and feedback. Similarly, when Paschalidis sets out to improve, say, a network of sensors, he and his research team write computer software that takes in data about how the system is working and then finds ways to correct or improve it.
In this project, hospital patients are the systems.
Fortunately, EHRs offer plenty of data—test results, diagnoses, prescriptions, emergency room (ER) visits, previous hospitalizations, demographic information. It’s far too much for doctors and nurses to comb through manually, but enough to feed an algorithm that automatically processes the information and flags at-risk patients. The software works by sifting through records of patients who were previously hospitalized and learning which risk factor—a certain number of chest complaints or an unusual level of a particular enzyme in the heart, for example—might have been red flags. The algorithm then uses those red flags to warn of future hospitalizations.
The challenge for Paschalidis was understanding how to properly use medical data and how to incorporate this kind of software in an actual hospital. That’s where Adams comes in.
A pediatrician and medical informatician (someone who uses information technology to improve health care), Adams has spent the past 20 years thinking about how to use data from EHRs to improve patients’ health outcomes, especially among families in Boston’s urban communities. He’s also one of the lead scientists at BU’s Clinical & Translational Science Institute (CTSI), one of 60 such sites across the country that aim to accelerate medical advances by encouraging researchers in disparate fields to collaborate on medical research.
“This is a perfect example of translational research collaboration,” Adams says. “Yannis and his lab have exceptional skills in data mining that we don’t have, but we have extraordinary data and clinical expertise.”
To use that data, Paschalidis and his team first needed a crash course in medical terminology to make sure they understood what they were working with. Much of EHR data is contained in a kind of “clinical language” that only doctors understand, Adams says. Sometimes, he says, even the same term can have different meanings, depending on the context in which the doctor records it. For example, a diagnosis of hypertension (high blood pressure) can be recorded as either a diagnosis made during a visit or a problem on the patient’s problem list. Both could be recorded with the same code (ICD-9 401.9), but users would need to know to look further to decide which of the two meanings the data represents. Cleaning up “messy” data—figuring out what it means, what to use, and how to represent it in the software—is time-consuming but important, Paschalidis says. “If you fit garbage to an algorithm,” he says, “you’ll get garbage as output.”
The researchers remove any identifying information from the EHRs using open-source software from a National Institutes of Health-funded center at Harvard University called i2b2 (Informatics for Integrating Biology & the Bedside).
Once the data is cleaned up and anonymized, Paschalidis and his graduate students can enter it into their software. The algorithm they built classifies patients as either at risk or not at risk for heart-related hospitalizations within one year. An elderly patient or someone who visited the ER in the previous year, for example, might be at risk, while a younger person who hasn’t been to the hospital in a few years might not be at risk. How the algorithm will ultimately present this information to doctors is still under development.
To test the software, Paschalidis and his students collected the EHRs of just over 45,500 patients from BMC. They used about 60 percent of the records to train their so-called machine learning software, teaching it which factors had put patients at risk for hospitalizations in the past. Then, they used the remaining data to test the software’s ability to make predictions. They found that it could correctly predict up to 82 percent of heart-related hospitalizations, while falsely predicting hospitalizations in about 30 percent of patients who weren’t actually at risk. Paschalidis says that it’s possible to reduce the number of false predictions, but doing so would correspondingly lower the number of accurate predictions. A false prediction rate of 10 percent, for example, would correspond to an accurate prediction rate of 65 percent.
“In medicine, we’re constantly trying to balance between something that’s concerning and something that might be a false positive,” Adams says. In many cases, however, the recommendations that would come of a false positive—healthy eating, exercise, an extra check-in with the doctor, extra visits from a nurse—could still benefit the patient. And, Paschalidis says, preventing hospital visits that each cost thousands of dollars is worth the occasional unnecessary checkup that only costs a couple hundred dollars.
Adams and Paschalidis published their findings about the machine learning software’s success in predicting heart-related hospitalizations in March 2015 in the International Journal of Medical Informatics. Their co-authors included Professor Venkatesh Saligrama (ECE, SE); Wuyang Dai and Theodora Brisimi, ENG PhD students working with Paschalidis; and Theofanie Mela, a cardiologist at Massachusetts General Hospital.
“If coupled with preventive interventions, our methods have the potential to prevent a significant number of hospitalizations by identifying patients at greatest risk and enhancing their patient care before they are hospitalized,” the researchers write in the study. “This can lead to better patient care, but also to substantial health care cost savings. In particular, if even a small fraction of the $30.8 billion spent annually on preventable hospitalizations can be realized in savings, this would offer significant results.”
Ultimately, Adams says, having this kind of ongoing, automated analysis within electronic medical records could not only help doctors, nurses, and case managers monitor their patients more effectively, it could also elucidate disease risk factors previously undetected by doctors.
“All of us know that a serious problem like diabetes is always going to increase your likelihood of being admitted to the hospital,” Adams says, “but the trick is to determine whether it’s about the thing that’s happening to your diabetes or something else unrelated to your diabetes that has substantially increased the likelihood of being hospitalized. The machine learning software has the potential to learn new associations.” These could be associations between some clinical features that make it more likely for the patient to develop serious complications from diabetes.
In the coming year, Paschalidis and Adams will be interviewing doctors, trying to figure out how best to put this kind of predictive software to work in an actual hospital.
“I’m confident that it will work,” Paschalidis says. “The issue is, what is the best way of incorporating something like that in the practice? Will the doctors use it or ignore it?”
Eventually, Paschalidis says, he’d like to expand the software to predict other, non-heart-related hospitalizations. He’s also currently working with BMC’s surgery department on software designed to flag patients at risk for readmission within 90 days, so hospitals could perhaps monitor those patients more closely. The 90-day window is of particular interest to hospitals because Medicare doesn’t reimburse for readmissions within that timeframe.
Down the road, Paschalidis says, it might also be possible to use data from wearable technologies in addition to EHR data. The data is there, he says; it’s just a matter of getting access to it.
“We carry these smartphones and now these smart watches and all of these fitness trackers and other devices that know much more than the hospital knows about our state of health,” he says. “You now have a much richer record about the patient, and the richer the record is, the better prediction you can make.”
Throughout his career, Paschalidis has put his data analysis skills to use in a lot of different areas. For the past three years, he’s been applying those skills to developing sensor networks for “smart cities.” He says he thinks he’ll be working in health care for a while.
“I feel that health care is an important area,” he says, “and the contributions that you make are somehow more tangible in terms of the potential outcome.”
The story was originally published at BU research and was highlighted as News from the Field by the National Science Foundation.