Smith, Rainie, Sheneiderman, and Himelboim (2014) identified six distinct types of community clusters on Twitter: Polarized Crowd, Tight Crowd, Brand Clusters, Community Clusters, Broadcast Network and Support Network. I am going to discuss examples of Broadcast Network and Tight Crowd through analysis of communities using #AdWeek (Broadcast Network) and #SFCyberSecurity (Tight Crowd).
First, the following is an example of a Broadcast Network around AdWeek and its #AdWeekChat. Ad Week Chat is a weekly article regarding brand marketing published by the website asking readers to share their opinions.
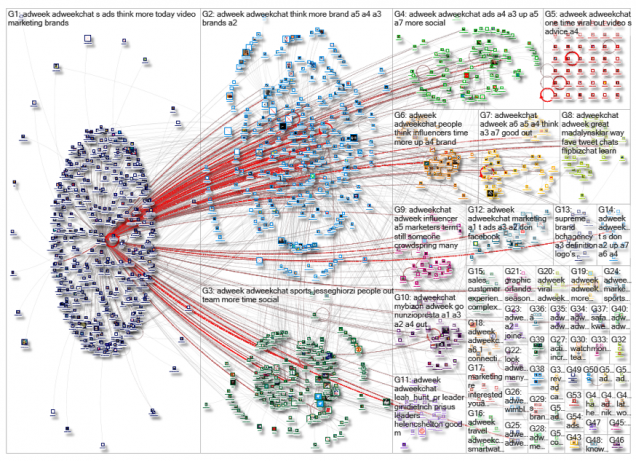
According to the Node XL visualisation, users react to the article using mostly #adweek or #adweekchat. This graph matches the description identified by Smith et.al. (2014). The @adweek handle is the media outlet with a distinctive hub, being the most connected to other users in all of the remaining groups. The hub audience is connected dominantly only to the source hub, rather than each other. This graph supports the point of Smith et.al (2014), that there are still strong agenda-setting players in the social media world, including media sources with large numbers of followers. There is a large audience that is connected only to @adweek in Group1, while Groups 2, 3 and 4 demonstrate audiences that are connecting with each other, in addition to connecting with @adweek. This means they are members of different, denser communities according to Smith et.al (2014). Group 5 also matches Smith’s et.al (2104) description, as there are isolated members, which suggests that the topic was visible to a wider audience even though they were not actively connecting with @adweek or other users. The most used hashtags are #adweekchat, #justdoit, #worldcup and #primeday. This suggest that users are responding to @adweek and commenting on the mentioned specific topics. This type of communication matches Smith’s et.al (2014) classification of a broadcast network, as most users start with referencing the broadcaster rather than communicating amongst each other. After @adweek, @dangerinaskirt and @chargegoforward are the most replied-to users in Groups 1,2, 3 and 4, showing they have consistently the biggest influence in this conversation.
This structure demonstrates how social media changed the landscape of communication with traditional media through transforming reader engagement by inviting “challenge, amplification, and adjustment by users of the news site” (Rainie & Wellman, 2012, loc. 5969). In this sense, although the impulse comes from traditional media outlet, the social media users are network creators collaborating on and directing the agenda. Although @adweek is in the center of the information flow and there are fewer connections between other users, several other users rise as influencers in connected smaller communities, such as in Group 2 and 3 in this example. Thus, a traditional medium sets the agenda but conversations also flow between other influencers and their connections creating a wider discussion leading into networked content creation described by Rainie and Wellman. In addition, these secondary influencers can use connecting to the main source in order to increase their visibility, advertise themselves and build networks with strangers feeding of a more popular outlet (Rainie, Wellman, 2012, loc.5640).
The second graph is an example of a Tight Crowd. Smith et. Al (2014) characterise Tight Crowd as “highly interconnected people with few isolated participants”. This graph shows users engaging in communication regarding the SF Cyber Talks Conference, a conference for the leadership in cyber security in San Francisco.
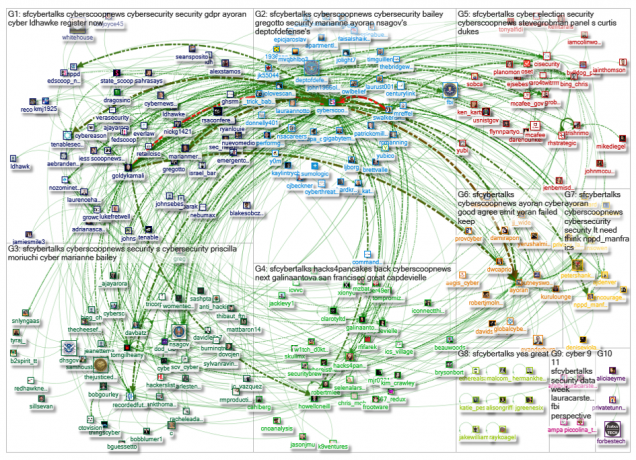
The graph matches the Tight Crowd description, as majority of the members have at least one connection, with some users being more active than others. This tight crowd graph includes some isolated users, which according to Smith et.al (2014) means that this event is reaching new communities of people. This conversation is divided into several subgroups that have many inter-group connections, which leads to the creation of more closely tied communities matching Smith’s et.al (2014) description. The 230 Twitter users in this graph all used #SFcybertalks, replied to or were mentioned in these Tweets. The top mentioned influencer is @cyberscoopnews, the organiser of the conference, while the top replied to is @ayoran, the CEO of Tenable Security and one of the speakers. Users in this graph are likely to retweet and reply to each other, while mentioning the name of the conference. Even though they are divided into smaller communities, their strong inter-connectedness qualifies them as a Tight Crowd.
This graph also resembles the theory of networked creators described by Rainie and Wellman, specifically the “collaborative content” on sites like Wikipedia (Rainie & Wellman, 2012, loc.5355). The Wikipedia editors’ community isa closely tight one, collaborating with each other and having connections to multiple users regarding the same topic. Rainie & Wellman present a graph of users working on different articles regarding the Fukushima disaster, which is resembling the structure of the SF Cyber Talks graph mentioned above. As Rainie and Wellman suggest, “the graph [Fukushima editor network] shows interconnectivity of articles, ideas, and people,” (Rainie&Wellman, 2012, loc.5380) which is in accordance with the description of a Tight Crowd of Smith et.al (2014).
The online community classification by Smith et.al (2014) helps us visualise the transformation of media. Allowing anyone with an Internet connection to take part in online networked creation completely changes the dynamics of media, relationship between experts and amateurs and individual influence on agenda setting (Rainie&Wellman, 2012, loc.5734). Closely tied networked creators can exchange information or collaborate on creating content such as in the case of SF Cyber Talks or Wikipedia, while traditional media is directly influenced by input from online users such as in the case of Ad Week. Overall, Rainie & Wellman shake hands with Smith et.al. providing details on how different network structures are shaped by as well as shape the information world.
References:
Rainie, H., & Wellman, B. (2014). Networked: The new social operating system. Cambridge, MA: MIT Press. Kindle Edition.
Smith, M. A., Rainie, L., Shneiderman, B., & Himelboim, I. (2014, February 21). Mapping Twitter Topic Networks: From Polarized Crowds to Community Clusters | Pew Research Center. Online.