Artificial Intelligence for Alzheimer’s Disease (AI4AD)
Project Title: Ultrascale Machine Learning to Empower Discovery in Alzheimer’s Disease Biobanks
Project Goals: The goal of this proposal is to develop transformative AI approaches for high throughput analysis of next generation sequencing (NGS) and related AD biomarker and cognitive data. Our collective effort in this proposal will sieve extensive genomic, biomarker, and cognitive data to extract and prioritize the features that are essential to address fundamental barriers to AD prevention and drug discovery.
Project Website: http://ai4ad.org
Project Summary: Alzheimer’s disease (AD) is a progressive neurodegenerative disease with complicated underlying disease mechanisms. About 99% of clinical trials for Alzheimer’s disease have failed after 2003. The failed AD trials are largely responsible for heterogeneity of participants in clinical trials. This observation may indicate that AD is multifactorial disease with complex etiologies leading to multiple forms of clinical and neuropathological manifestations. Therefore, there is an urgent need to develop multiple treatment options depending on a patient’s underlying disease mechanism. Precision medicine is to develop patient driven treatment solutions, largely depending on genetic profiles and the availability of existing drug options. Precision medicine practice is recently common and successful in cancer but are largely unexplored in AD. A highly collaborative team spanning 11 institutes has been assembled to investigate extensive genomic, biomarker, and cognitive data to extract and prioritize the features that are essential to address fundamental barriers to AD prevention and drug discovery. By leveraging the power of artificial intelligence (AI) and machine learning (ML), the parent application will develop a suite of complementary big data analytic approaches for ultra-scale analysis of AD genomic and phenotypic data for more precise characterization and early detection of AD.
Dr. Jun will be the Director of Genome Guided Drug Discovery Core at the AI4AD initiative. Dr. Jun’s team is to prioritize genetic variants identified in this project and determine network-based signatures for repositioning existing drugs in AD therapeutics. Recent extended efforts by Dr. Jun’s lab is to enable precision medicine concepts with AI/ML applications on target prioritization and drug repurposing in AD therapeutics. Dr. Jun’s lab is to develop an AI/ML-ready database by profiling predictor, signature, biomarker, and outcome (PreSiBO) features for targets and existing drugs applicable to the corresponding genome guided patient subgroups. The database will facilitate scalable and efficient evaluation of targets and existing drugs using AI/ML tools developed by the parent application, as well as improve collaborations between interdisciplinary investigators from the parent application. This extended effort will increase our readiness of AI/ML applications in precision medicine for AD prevention and treatment.
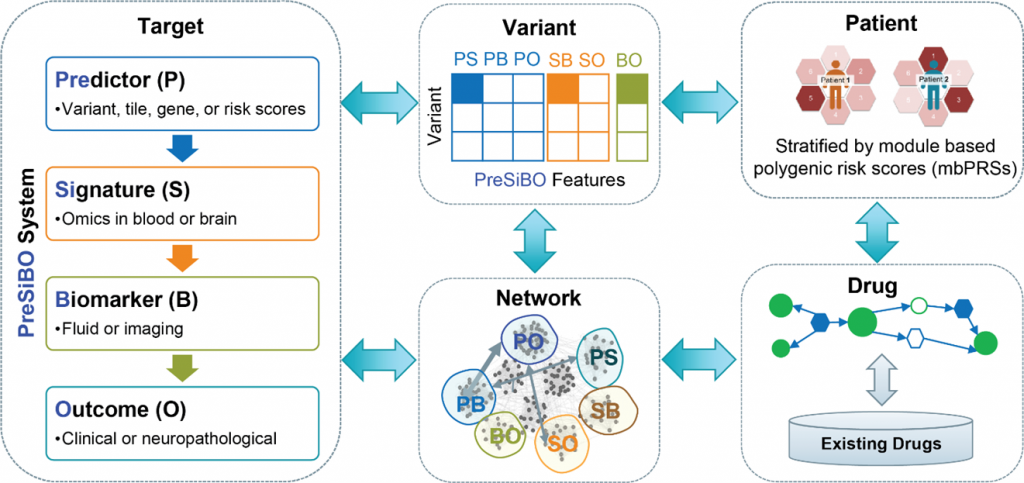